What Is ChatGPT Vision? Best Ways People Are Using This Feature
This article will dive in and discover how ChatGPT Vision works and what people are using it for!
What Is the Vision Feature in ChatGPT?
ChatGPT Vision is a feature that enables ChatGPT to interpret and respond to visual content. Specifically, ChatGPT Vision uses its newfound “sight” to analyze images and connect them to your text prompts. It can describe what ChatGPT sees in a photo, answer your questions about it, and even generate creative text formats inspired by the image.
ChatGPT Vision is a feature exclusive to ChatGPT Plus, the premium version of ChatGPT. The free tier of ChatGPT does not include access to this functionality.
Here’re some examples you can do with ChatGPT Vision:
- You show ChatGPT a picture of your dream vacation spot and get itinerary suggestions.
- You describe a clothing style and receive outfit recommendations with visuals.
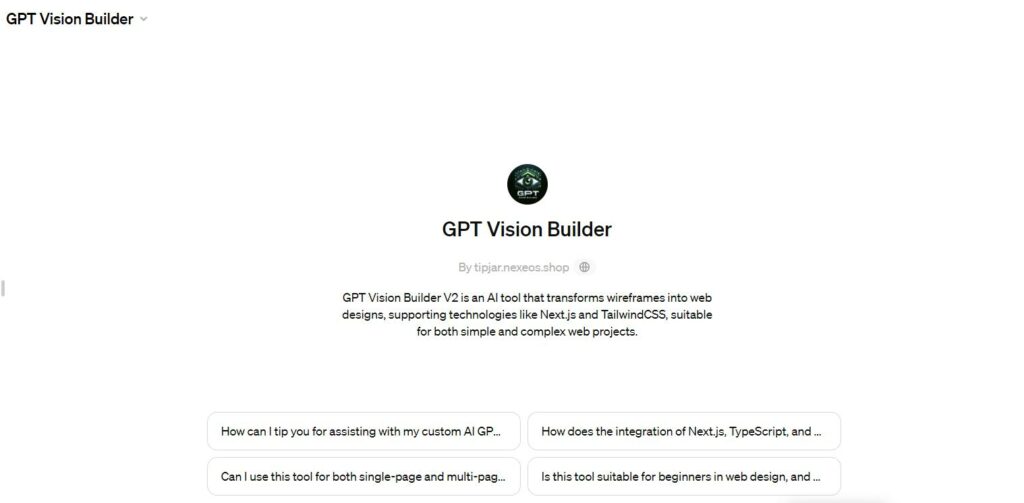
How Does ChatGPT Vision Work?
Here is a step-by-step breakdown of how ChatGPT Vision works.
Step 1: Input Collection
First, ChatGPT Vision collects visual data, which could include still images or video streams.
This visual data serves as the raw material for the AI to analyze.
The sources of these images can vary widely, from user-uploaded photos to live feeds from cameras. They provide a broad range of contexts and scenarios for the AI to interpret.
Step 2: Preprocessing
Once an image is inputted, it undergoes preprocessing.
This step involves resizing the image to a uniform scale, adjusting lighting conditions, or normalizing colors.
The purpose is to reduce variability in the visual data that could confuse the model. Preprocessing ensures that the AI’s analysis is as efficient and accurate as possible, similar to tuning an instrument before a performance.
Step 3: Feature Extraction
The preprocessed images are then passed through algorithms designed to extract features. These features include edges, textures, color distributions, and more.
Typically, convolutional neural networks (CNNs) are used for this purpose. CNNs are adept at hierarchically extracting and learning from visual features. This ability allows them to understand complex patterns in the data that are crucial for the next stages of analysis.
Step 4: Model Training
Feature-rich data is fed into machine learning models – CNNs.
Sometimes, it’s more complex architectures like transformers if the task involves understanding spatial relationships across a series of images.
These models learn from huge collections of labeled images. During training, they adjust their settings to get better at making predictions, like identifying objects or spotting unusual things. They learn what the right answers should be by practicing with each example.
Step 5: Inference
Once trained, the model can make predictions on new, unseen images.
This process, known as inference, involves the model using its learned knowledge to interpret and analyze images.
The output varies based on the application. It could be identifying objects. Or categorizing images into different classes. It might even generate descriptions of scenes in the images. This step shows how the AI uses its learning in real-world scenarios.
Step 6: Post-Processing and Output
The raw output from the inference stage often goes through post-processing.
This step enhances its usefulness or clarity. It might involve converting probability scores into clear categories or adding boxes around objects in images. It could include creating text descriptions.
The final output is tailored to fit the specific needs of different applications, making it readily usable. They aid in medical diagnoses and boost surveillance systems. They improve interactions in consumer apps. They even help steer autonomous vehicles. This expands how AI can help in daily tasks.
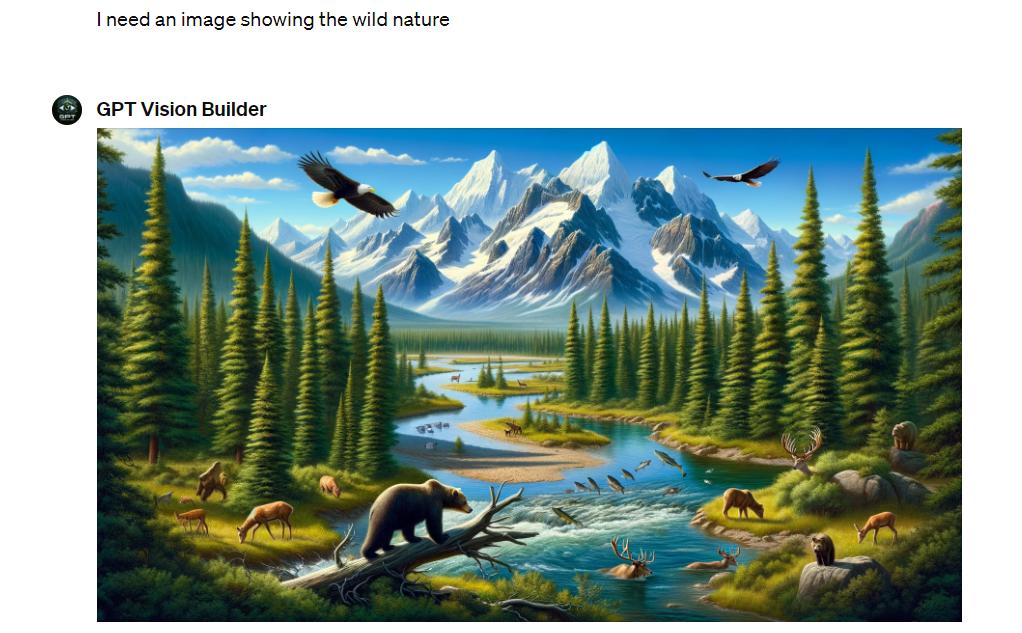
How Do I Access ChatGPT Vision?
Gaining access to ChatGPT Vision is easier than you might think. Below is a simple guide on how to get started, whether you’re a developer, a business, or just curious about the possibilities of AI vision technology.
Step 1: Log In to Your Plus Account
To access and use the ChatGPT Vision feature, ensure you log in with a ChatGPT Plus account. This advanced capability is exclusive to the premium tier, available only to Plus subscribers.
Step 2: Navigate to the Vision Feature
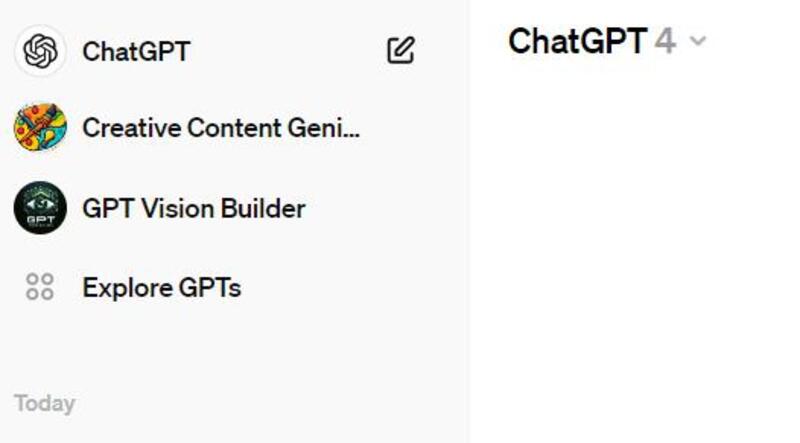
Once you’re logged in, navigate to the ChatGPT Vision feature. This is located in the main menu or as a separate tab on the website.
You look for a section specifically dedicated to image analysis or visual understanding. Click on it to access the Vision feature and proceed to the next step.
Step 3: Upload or Provide Images
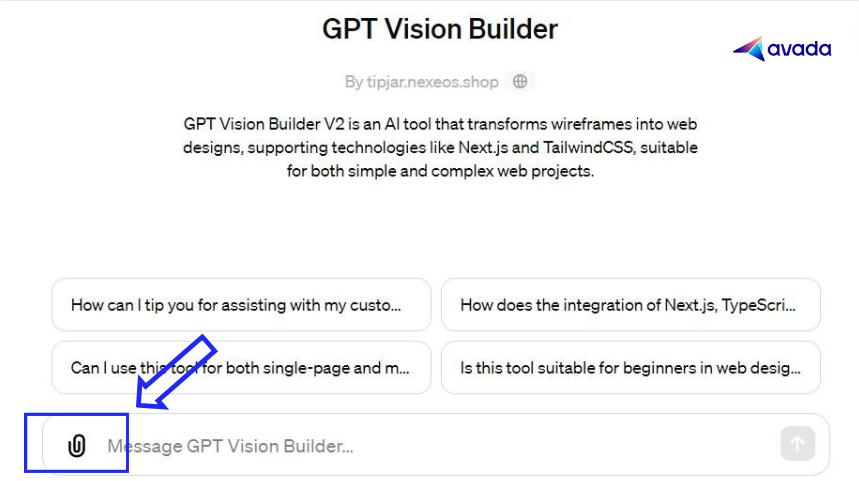
In the Vision feature, you will be given options to upload images for analysis. Click on the “Upload” button and select the images you want to analyze from your device’s storage. Alternatively, you may have the option to provide image URLs or specify the source of the images. To finish this step, simply follow the instructions displayed on your screen.
Step 4: Initiate Image Analysis
After uploading or providing the images, initiate the analysis process. The AI-powered algorithms and models of ChatGPT Vision will start processing the images, extracting features, and providing insights and interpretations based on the visual content.
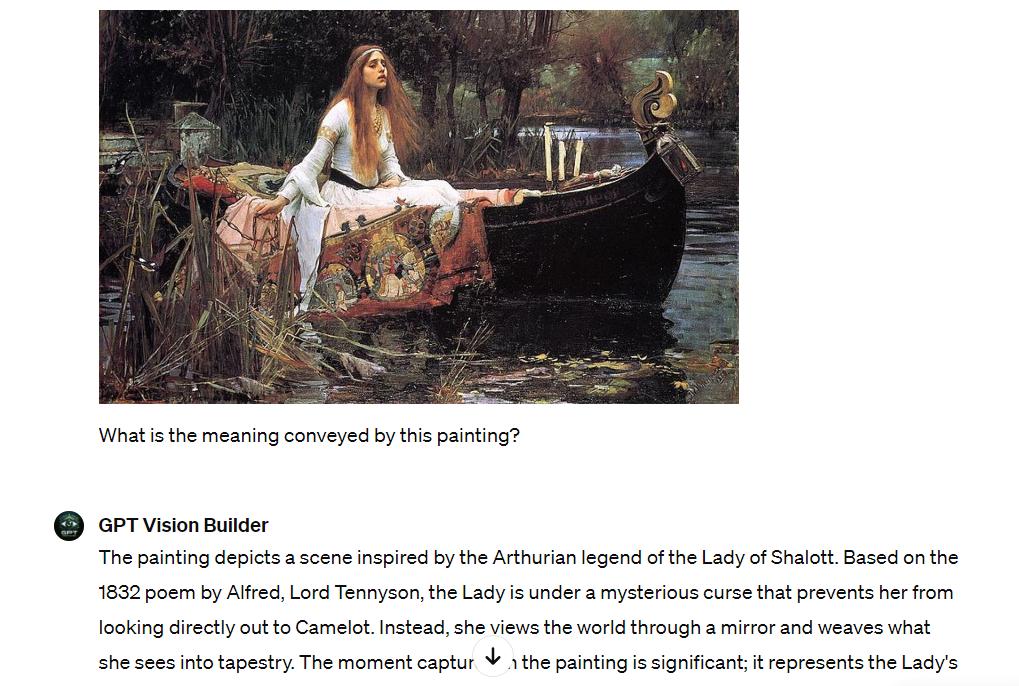
Step 5: Review and Interpret Results
Once the analysis has concluded, the results will be displayed to you. These may include identified objects, categorized scenes, or descriptive texts about the images. Take your time to review and interpret the results provided by ChatGPT Vision. This can help you gain valuable insights and make informed decisions based on the visual information analyzed.
Step 6: Repeat or Explore Further
If you have more images to analyze or wish to explore additional features of ChatGPT Vision, simply repeat the process by uploading or providing new images. You can continue to leverage the power of AI-driven image analysis to extract meaningful information from a wide range of visual content.
Why ChatGPT Vision is Different?
ChatGPT Vision is a revolutionary new feature that’s changing the way we interact with language models. Unlike traditional chatbots, ChatGPT Vision can not only understand our words, but also see the world through our eyes. This powerful combination unlocks a whole new level of communication and assistance.
Let’s take a look at the top reasons why ChatGPT Vision is a standout choice.
Advanced Integration of Natural Language Processing
ChatGPT Vision uniquely integrates advanced natural language processing (NLP). This allows the system to not only see images but understand and describe them in human-like language.
For instance, it can analyze a scene in a photo and discuss it as a person might, recognizing emotions, actions, and more. This capability makes it incredibly powerful for applications requiring deep understanding, such as aiding in educational tools or enhancing interactive customer support.
See What You Mean
ChatGPT Vision can interpret images based on context and intention behind user queries. If you ask, “Show me the happiest moment,” it can analyze videos or pictures and identify those that depict happiness. This understanding goes beyond literal seeing. It’s about perceiving the intent behind your words, making the interaction much more meaningful and personalized.
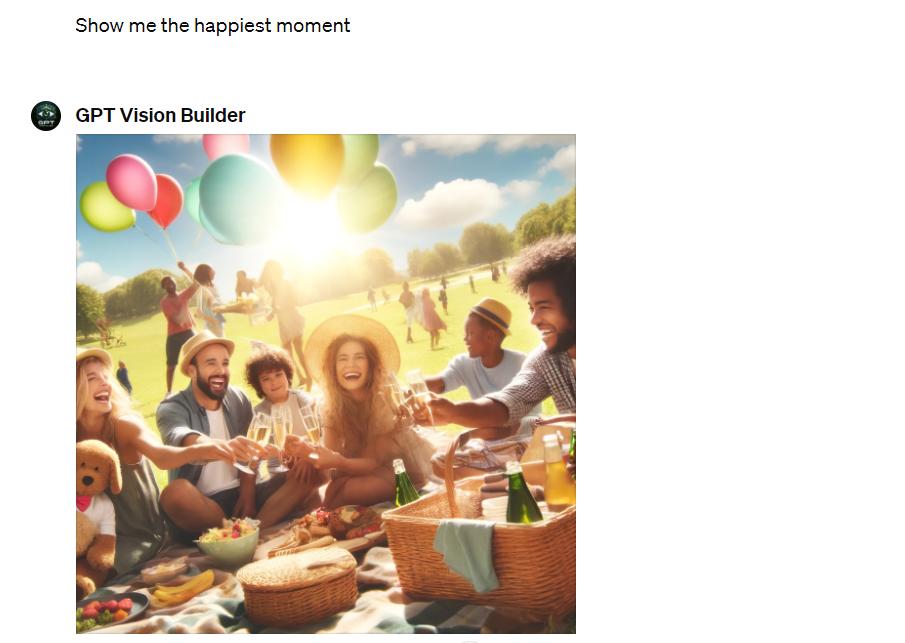
Break Down Language Barriers
ChatGPT Vision breaks language barriers. It understands and interprets visual content in different languages. If you ask a question in one language, it can provide answers or descriptions in another language.
This is useful in global applications, like social media or international news, where visual content needs to be quickly understood and communicated across diverse language groups.
Accessibility for All
ChatGPT Vision is a powerful tool for people with visual impairments. Features like image description for the visually impaired or sign language detection from videos make digital content more accessible.
Additionally, its ability to interpret and interact with users through simple language makes technology easier for everyone to use, regardless of their tech-savviness. This commitment to inclusivity not only expands the user base but also greatly enhances the user experience.
How to Use GPT-4V
ChatGPT Vision, or GPT-4V, is here to revolutionize the way you interact with information. This guide will equip you with the knowledge and tips to get the most out of GPT-4V.
Guide for Users
1. Accessing GPT-4V
- First, ensure you have access to a platform that supports GPT-4V.
- Sign up for an account if required, and log in to start using the service.
2. Setting Up:
- Depending on your platform, you might need to configure certain settings to tailor the experience to your needs.
- This could involve adjusting privacy settings, choosing language preferences, or selecting specific features you want to enable.
3. Uploading Content:
- GPT-4V typically requires input in the form of images or videos. Use the platform’s interface to upload this content.
- Ensure that the visuals are clear and the relevant subjects are easily identifiable to maximize the model’s accuracy.
4. Interacting with GPT-4V:
- Once your content is uploaded, you can start interacting with GPT-4V.
- You can ask specific questions about the image or request descriptions and analyses. For instance, you can inquire, “What is happening in this image?” or “Could you describe the emotions portrayed in this picture?”
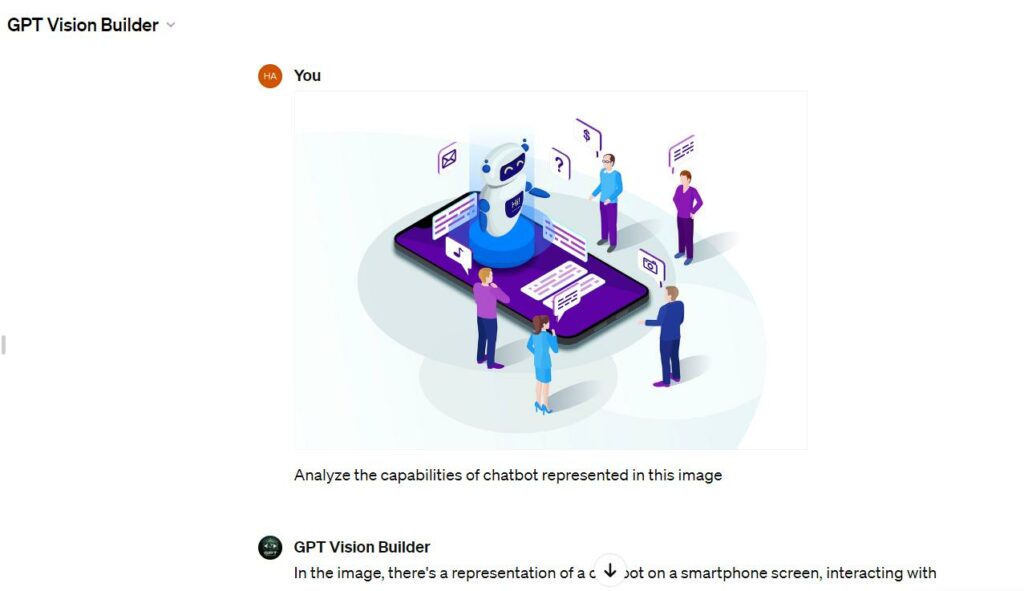
5. Receiving Outputs:
- GPT-4V processes your requests and provides outputs based on the visual and textual data it analyzes.
- The responses can be textual descriptions, tags, annotations, or even detailed reports, depending on what you asked for.
Tips for Optimizing the Experience with ChatGPT Vision
- Use high-quality images: The clearer your images, the better GPT-4V can perform. High-resolution images with good lighting conditions help the model analyze details more accurately.
- Be specific with queries: The more specific your questions, the more accurate and relevant the responses will be. If you need detailed information, guide the model with clear and concise questions.
- Explore various features: Don’t limit yourself to basic functions. Let’s explore the different capabilities of GPT-4V. You can try out features like comparative analysis, trend identification, or even creative generation based on the images you provide.
- Provide feedback: Many platforms allow you to provide feedback on the AI’s performance. Use this feature to help improve the model. Feedback on accuracy, relevance, and usefulness of the outputs can guide future updates.
- Stay updated: Keep your application or platform updated to benefit from the latest improvements and features added to GPT-4V. Updates may include enhanced understanding, faster processing times, and new functionalities.
Are Multimodal LLMS the Future of AI?
Yes. AI is constantly evolving, and Multimodal Large Language Models (MLLMs) are a major driving force in its future. We first need to understand what they are, how they compare to existing LLMs, and what the future might hold for them.
Mistakes and Limitations

- Data biases
AI systems learn from data. If that data is biased, the AI’s outputs will also be biased. This can reinforce existing societal biases. For example, a facial recognition system trained mostly on images of a particular race may perform poorly on others. This can lead to unfair treatment, affecting minorities disproportionately.
- Explainability challenge
AI’s decision-making process can be a “black box.” Algorithms make decisions in ways that are difficult for humans to interpret. This lack of transparency complicates understanding how AI reaches certain conclusions. In critical applications like medical diagnosis or credit scoring, this can lead to mistrust or flawed outcomes without clear justification.
- Misinformation and deep fakes
AI can generate realistic but fake content, known as deep fakes, which can spread misinformation. This technology can create false representations of people, which can be particularly damaging in political contexts, personal reputations, or public trust.
The ability of AI to produce convincing fake news and media poses a serious challenge to information integrity and media authenticity.
- Security risks
AI systems are susceptible to cyber attacks that manipulate their behavior, leading to compromised functionality. Adversarial attacks involve subtly altering input data to fool AI models into making wrong decisions. Such vulnerabilities threaten the security of the AI system. They can have broader implications, risking personal and organizational safety.
What to do to mitigate the risks
- Diverse datasets: Training MLLMs on comprehensive and diverse datasets is crucial to minimize bias.
- Explainable AI (XAI) techniques: Developing methods to understand how MLLMs reach their conclusions fosters trust and allows for error correction.
- Fact-checking and verification systems: Implementing robust fact-checking mechanisms can help combat the spread of misinformation generated by MLLMs.
- Security safeguards: Building strong security measures into MLLM development can minimize the risk of malicious exploitation.
3 Possibilities ChatGPT Vision Opens
ChatGPT Vision unlocks a treasure trove of possibilities. From revolutionizing education to unlocking deeper data analysis, its impact promises to be profound.
Revolutionizing Education
ChatGPT Vision can fundamentally change the way educational content is delivered and interacted with. Learning will become more immersive and tailored to individual needs.
- Bringing history to life
Example: A history class where students aren’t just confined to textbooks. With ChatGPT Vision, historical figures can come alive through analyzed portraits.
Students can ask questions about the clothing, expressions, and objects depicted, prompting ChatGPT Vision to provide historical context and cultural insights. This can spark deeper discussions and a more immersive understanding of the past.
- Interactive learning environments
ChatGPT Vision can create dynamic learning experiences that cater to diverse learning styles. Students can analyze primary source documents like historical maps.
By prompting ChatGPT Vision, they can translate handwritten text, highlight key geographical features, and even receive summaries of historical events related to specific locations. This interactive exploration fosters a deeper understanding of the subject matter.
- Personalized learning paths
ChatGPT Vision can empower educators to create customized learning materials. Teachers can upload images and prompt ChatGPT Vision to generate quizzes, flashcards, or even personalized study guides based on individual student needs and learning gaps. This data-driven approach allows for a more effective and targeted learning experience.
SEO and Content Creation
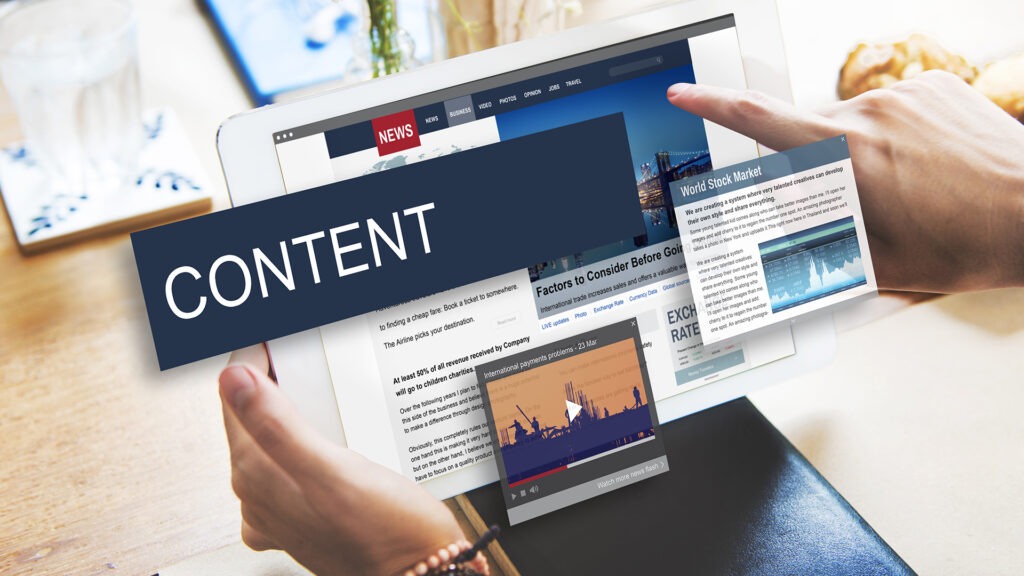
ChatGPT Vision isn’t just for students; it can also empower content creators and marketers:
- Image optimization
ChatGPT can analyze images and suggest relevant keywords, alt tags, or captions to optimize them for search engines. This helps content creators improve the discoverability and visibility of their visual content, driving more traffic to their websites.
- Visual content generation
ChatGPT’s visual understanding can generate engaging visual content, such as infographics or illustrations, based on textual prompts.
Content creators can provide a brief description or outline. ChatGPT can create visually appealing assets that align with the desired message or theme. Therefore, it saves time and effort in content creation.
- Content enhancement
ChatGPT’s visual understanding can provide suggestions to improve the visual appeal of articles, blog posts, or social media content.
It can offer ideas for incorporating relevant images or videos to enhance the overall user experience, making the content more engaging and shareable.
Deeper Data Analysis
ChatGPT’s visual understanding capabilities open up new possibilities for in-depth data analysis. This leads to valuable insights and more informed decision-making.
- Social Media monitoring
ChatGPT can analyze visual content from social media, like images or videos. It extracts valuable information. This helps businesses track brand mentions and analyze sentiment. They can also identify emerging trends from visual cues. This allows businesses to adjust their marketing strategies effectively.
- Market research
ChatGPT’s visual skills assist in market research. It can analyze product images or customer reviews. This helps identify patterns, preferences, or sentiments related to products or brands. These insights are valuable. Businesses can use them to make data-driven decisions and improve their offerings.
- Real-time surveillance analysis
For security, ChatGPT Vision can analyze surveillance camera footage. It detects unusual activities or identifies items of interest in real-time. This improves security measures and response strategies. It is especially useful in public spaces and sensitive environments.
- Medical image analysis
In healthcare, ChatGPT Vision can analyze medical images, such as MRIs or CT scans. It uses these alongside patient records. This helps diagnose diseases earlier and more accurately. It supports doctors in making better-informed decisions.
In summary, integrating visual understanding with ChatGPT opens revolutionary possibilities. These impact education, SEO, content creation, and data analysis.
GPT-4V gives learners interactive and personalized experiences. It helps content creators optimize and enhance visual content. Businesses gain valuable insights from visual data. The impact of these advancements could reshape industries.
GPT-4 Vision vs. DALL-E 2/DALL-E 3
DALL-E is a family of AI models developed by OpenAI, specializing in generating images from textual descriptions. DALL-E 2 was the first iteration, known for its ability to create realistic and detailed images based on user prompts. DALL-E 3, the latest version, boasts even greater capabilities, generating even more impressive and creative visuals.
While DALL-E 2 and DALL-E 3 specialize in generating highly detailed images from textual descriptions, GPT-4 Vision is designed to be more interactive and versatile. It not only generates images but also comprehends and responds to images provided by users.
The table below will clarify the differences between ChatGPT Vision and DALL-E:
Feature | ChatGPT Vision | DALL-E 2/DALL-E 3 |
Primary Function | Understand existing images and enhance text-based interactions | Generate images from text descriptions |
User Input | Text prompt + Image | Text prompt only |
Output | Text descriptions, answers, creative text formats (poems, stories) | Images |
Image Understanding | Yes, analyzes objects, scenes, and actions within the uploaded image | No, relies solely on the text description for image generation |
Strength | Enhances communication and problem-solving by leveraging existing visuals | Creates highly detailed and creative images based on user imagination |
Weakness | May not always perfectly interpret complex images. Creative outputs might require human editing. | May require complex and specific text descriptions to achieve desired results. Limited control over image style. |
Overall Focus | Empowering text-based interaction with visual information | Generating creative and novel images from scratch |
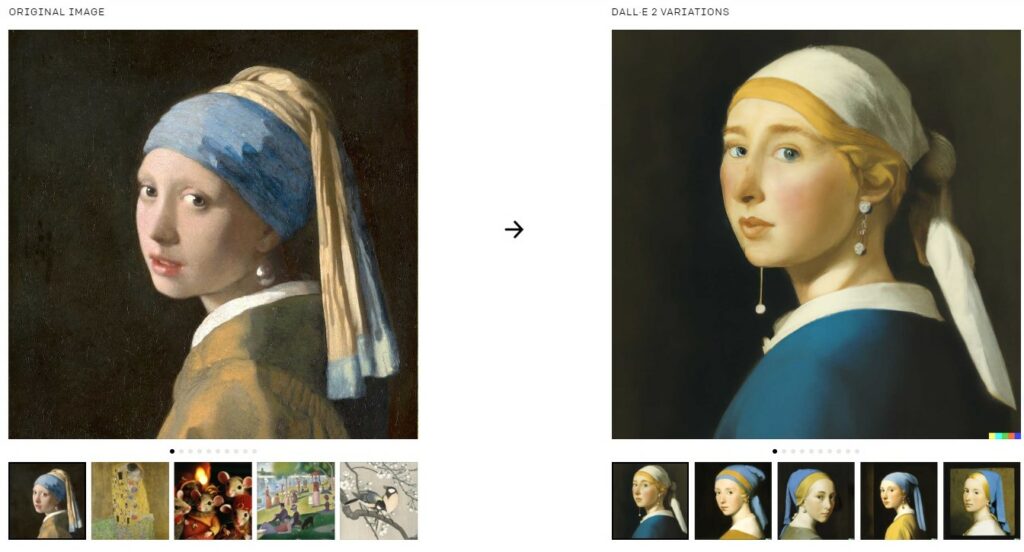
How Did OpenAI Build GPT-4V?
Let’s delve into the research and development process, the technological hurdles OpenAI faced, and the collaborative efforts that brought GPT-4V to life.

Research and Development Process
OpenAI’s journey with GPT-4V began long before the model itself. Years of research went into developing the foundational technologies that power GPT-4V, particularly in two areas:
- Natural Language Processing (NLP): This field focuses on a machine’s ability to understand and process human language. OpenAI has made significant contributions to NLP through its work on previous GPT models. Each iteration refines the model’s ability to grasp complexities of human language.
- Computer Vision: This area deals with a machine’s ability to interpret and understand visual information. OpenAI invested heavily in developing robust computer vision algorithms capable of recognizing objects, scenes, and subtle details within images.
With these core technologies in place, OpenAI embarked on the ambitious project of combining them. This meant bridging the gap between two distinct fields: the world of text and the world of images. The challenge lay in creating a model that could not only process language but also use that understanding to interpret visual data.
Technological Challenges and Breakthroughs
Building a robust multimodal model posed challenges. Integration between NLP and CV components needed to be seamless. Researchers bridged the gap between text and image data, enabling simultaneous processing. Novel training techniques and tailored architectures were developed for multimodal learning.
Another challenge involved data quality and quantity. Training a model as complex as GPT-4V requires massive amounts of data, both text and images. OpenAI had to curate and clean vast datasets. They ensured representation of the real world and avoided biases in the model’s outputs.
Breakthroughs came through innovative approaches. Transfer learning accelerated GPT-4V’s training using knowledge from pre-trained models. Self-supervised learning allowed the model to learn from unlabeled data, enhancing its understanding.
Collaboration and Partnerships in Development
OpenAI didn’t build GPT-4V in isolation. Collaboration played a vital role in the model’s success. OpenAI often collaborates with academic institutions, technology companies, and industry experts to enhance its R&D capabilities:
- Academic partnerships: OpenAI teamed up with top research institutions. They brought expertise in NLP, computer vision, and machine learning. This mix of knowledge sped up the development of core technologies.
- Tech industry collaboration: Working with tech companies gave OpenAI access to vast computing resources. These resources are essential for training a complex model like GPT-4V. This collaboration fostered exploration of real-world applications for models.
- Open source community: OpenAI’s commitment to open-source research principles allowed them to tap into the collective intelligence of the broader AI community. Shared research findings and code contributions from developers worldwide accelerated progress.
The development of GPT-4V shows the strength of focused research, innovative problem-solving, and teamwork. By combining different areas of expertise and addressing tough challenges, OpenAI has started a new era in how humans and computers interact. They are paving the way for a future where language and images come together.
Conclusion
As ChatGPT Vision continues to evolve, its impact on various industries and personal applications is increasingly significant. This article has outlined noticeable uses and the transformative potential of this technology. Embrace the future by exploring how ChatGPT Vision can be integrated into your daily life, opening up a world of possibilities.
FAQs: ChatGPT Vision
What is ChatGPT in computer vision?
How can I use ChatGPT 4 vision?
What are the capabilities of GPT-4 vision?
Can GPT-4 create visuals?
What is the goal of GPT?
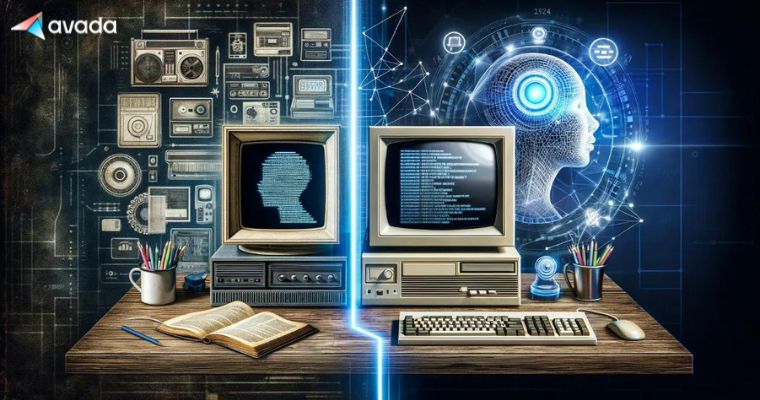
ChatGPT’s Second Anniversary

How to Use Kayak ChatGPT Plugin to Plan Your Trip
